The provision of generative AI in universities: What is possible and what is desirable?
The provision of generative AI in universities: What is possible and what is desirable?
28.02.24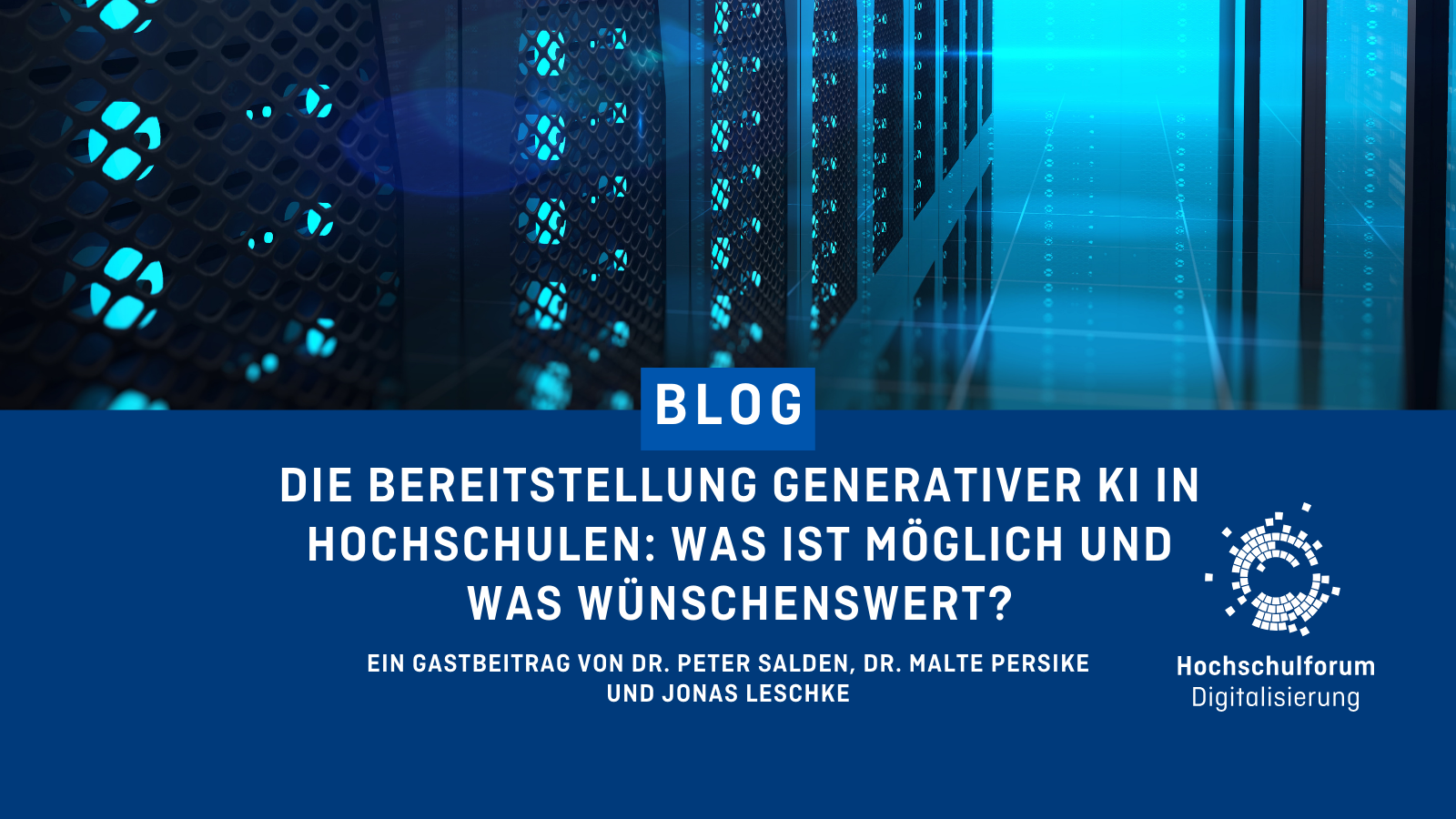
German universities are facing the challenge of making tools based on generative artificial intelligence (AI) accessible for research, teaching and administration. The focus is currently on the accessibility of commercially available tools such as ChatGPT. However, the issue of non-commercial, open source-based solutions is also increasingly coming to the fore in the discussions. What options are there, what are the advantages and disadvantages and what role does cross-university cooperation play here?
In this article, Dr. Peter Salden, Dr. Malte Persike and Jonas Leschke provide an overview and classify the extent to which non-commercial, open source-based applications can be a useful alternative at universities.
Generative AI: an ongoing topic for universities from now on
Since the publication of ChatGPT in November 2022, generative artificial intelligence (AI) has arrived in all areas of universities. The first year after publication was characterized by discussions about the risks and potential of the new technology. It is now clear that generative AI and applications based on it will also be used in universities in the long term. This applies at all levels – in studies and teaching, in research and in administration.
- Studying and TeachingStudents are already the group that uses generative AI comparatively intensively, for example to clarify questions of understanding, for literature research, for translations and to analyze, process and create scientific texts, as a study by Darmstadt University of Applied Sciences shows. This is another reason why it is important that the use of tools is addressed in teaching and ideally also learned in practice. Generative AI must therefore be able to be used for teaching and learning purposes in a legally compliant manner – and in such a way that the quality of the tools used does not depend on your personal wallet (keyword: educational equity).
- Research: In their Statement on the influence of generative models in science the German Research Foundation (DFG) has taken the position that generative AI can certainly be used for scientific work. The fact that this is already happening was made strikingly clear by the journal “Nature” when it recently, in connection with the award of the ten most influential scientists of the world also awarded an eleventh place – to ChatGPT. Researchers therefore need access to generative AI not only because it can be an object of research for them. In fact, it is likely to be a tool for any research work in all subjects in the future.
- Administration: Interest in generative AI is also growing in administration. This involves more than just support in the creation of forms and notes: chatbots based on generative AI can be equipped with concrete factual knowledge to reduce the likelihood of the much-discussed hallucinations or confabulations. These so-called “embeddings” make it possible to expand the AI with the university’s own material, for example to answer recurring questions in the student advisory service or to enable efficient interaction with the content of administrative documents.
The status quo: individual provision and use
The greater the awareness of the relevance of generative AI for universities, the more urgent the question becomes as to how the technology or applications based on it can be made available to students, teachers, researchers and the administration in a legally compliant manner and without individual costs. Most universities are currently improvising at this point: sometimes they refer to free offers for use, sometimes they pay the usage fees for paid tools for selected employees, sometimes computers with relevant programs are made available in libraries. In many cases, employees or students purchase licenses at their own expense in order to be able to use the full range of functions of the tools.
These approaches are not sustainable in the long term, as problems arise at different levels. With free tools in particular, the input data is often used for different purposes. With other tools, personal data such as a telephone number must be provided before use. Private costs are also incompatible with the idea of equal opportunities. Last but not least, the purchase of a large number of individual licenses does not appear to be the best option from a cost perspective either.
What to do? The answer to this question is by no means simple, as there are different ways of providing generative AI that are compliant with data protection regulations, legally unobjectionable and can be implemented sustainably.
Cooperation with commercial providers
With regard to generative AI, the offerings of the American company OpenAI, whose AI tools run on an Azure-based supercomputing platform from Microsoft, continue to be particularly popular. Azure-based supercomputing platform from the company Microsoft be executed. Like other AI providers, OpenAI makes its own language models accessible via an application programming interface (API). Simply put, this allows an institution – including universities – to place its own entry page (a web interface) between users and a service such as ChatGPT, whereby the personal login takes place on this entry page – not only at ChatGPT. The institutions assume the status of a normal “paying customer” who pays for a user license by credit card.
A major advantage of this solution is that personal login details and other personal metadata are not passed on – only the prompt from the institution is sent to the AI provider’s servers. The AI providers therefore never find out which individual has made a request to ChatGPT. In addition, this solution (as with individual payment accounts) can prevent user input data from being reused by the provider, for example for training the AI model. In many cases, however, the user experience for the user is sometimes significantly impaired, for example by no chat history is available.
Access to ChatGPT directly via OpenAI or indirectly via Microsoft differs in the location of the servers on which the AI models process their data. Data exchanged with OpenAI can also be processed on Azure servers in non-EU countries, while data processing for the AI models offered by Microsoft can be restricted to the EU.
The first data protection experts consider the use of large-language models via the API to be acceptable if the universities’ terms of use provide clear guidelines for data protection-compliant use of the services, e.g. by prohibiting the transfer of personal or otherwise sensitive data as part of prompts.
In the school sector, this has led to commercial providers such as fobizz setting up corresponding interfaces, with the institutions bearing the costs and then making the tools freely available to their users. Some universities are also in contact with corresponding providers. Unlike schools, however, universities generally have their own IT, which is why they are able to carry out the technically not particularly complicated API connection themselves (see, for example, the solution HAWKI).
However, this does not mean that this path is without stumbling blocks for universities. This is because clarification processes, including with data protection officers, staff councils and other bodies, as well as the provision of information for appropriate use, are required before the system can be put into operation. Above all, however, there is the question of costs, as the processing of chat requests by commercial providers is subject to a charge. Compared to traditional software licenses at universities, the conditions for this are unusual: usage is not charged at a flat rate or per user, but according to the volume of requests. The billing unit here is usually the so-called “token”, which is on average about three quarters of a word in English and thus corresponds to about half a German word. The more tokens are processed for a university, the more expensive it becomes. Even if extensive empirical values are still lacking, it is becoming apparent that the costs can rise into the five-digit range per month, especially for large universities. Most universities are therefore still taking the first steps cautiously, capping the maximum number of permitted tokens, limiting the group of authorized users or restricting access to the expensive (but also particularly powerful) models.
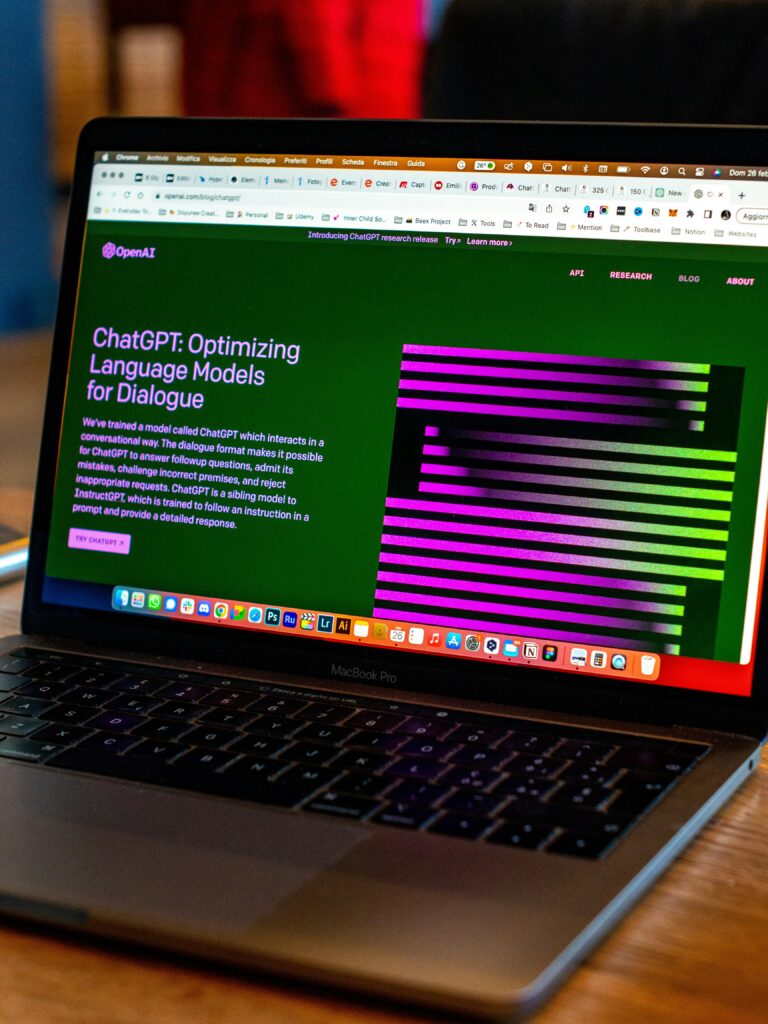
However, commercial solutions are also currently emerging in other ways. Microsoft, for example, has developed the so-called “Copilot 365” the OpenAI applications are integrated into the Office products that are also relevant for universities – however, these are generally not yet accessible at universities and are currently associated with considerable costs. Irrespective of this, Microsoft is currently approaching universities, not least in its role as an investor in OpenAI, in order to commercially exploit the applications.
It would also be conceivable to implement university-specific solutions in cooperation with commercial German providers. This is where the Cooperation between the state of Baden-Württemberg and the German language model provider AlephAlpha for the purposes of the Baden-Württemberg state administration. However, no interest in extensive cooperation between AlephAlpha or other companies and the education sector has been evident in the discussion so far.
In summary, it can be said that although the solutions offered by commercial providers of generative AI provide access to high-performance systems, they also pose different challenges in terms of costs, data protection and data security as well as educational equity.
Non-commercial solutions
The availability of non-commercial large language models raises the question of whether universities in Germany can provide generative AI themselves. Here is in particular open source solutions where universities keep the language models in their own hands and operate them on their own servers.
Could universities develop their own AI models for these purposes? The fact that German universities have internationally recognized expertise in this field is demonstrated by the example of the Stable Diffusion image program. In the field of text production, however, there are no signs of leading international open source models developed in Germany becoming available any time soon.
However, this does not mean that an open source solution is generally impossible. For example, existing open language models from international providers can serve as existing open language models from international providers, which are then stored on their own servers and optimized (e.g. retrained) for the purposes of the universities. Of such open solutions, the one currently available under a full open source license (Apache 2.0) published AI model Mistral from the French company Mistral AI and the open-source model “Llama 2” from Meta, which is licensed under the LLaMA 2 Community License (which imposes certain restrictions on further use).
The implementation of such models poses specific challenges: Firstly, it requires an advanced technical understanding of AI applications – no small feat given the shortage of IT specialists at many universities. In addition, considerable computing capacity is required in order to achieve satisfactory performance of the model even in the case of mass access by all university members. Last but not least, the open source models are likely to lag behind the quality of commercial solutions for some time to come, if not permanently.
From a data protection perspective in particular, however, the use of open source models that are as independent as possible would be desirable. Because even if – as mentioned above – the API solution described above can in part be regarded as theoretically compliant with data protection regulations, data is still ultimately processed on external servers. However, it is doubtful whether users always remember to keep sensitive data out of their prompts. The transfer of sensitive research data or the processing of data, for example from personnel departments and student advisory services, also appears to be (too) sensitive in the context of commercial services, so that self-operated open source applications would be advantageous.
Provision of generative AI: the example of North Rhine-Westphalia
The above statements also apply to the universities in North Rhine-Westphalia. Many universities are currently interested in implementing the API solution. At the same time, there is a growing awareness that cooperation is useful at this point, for example in the development of technical solutions, legal regulations and supporting documents. Especially under the umbrella of the Digital Universities NRW and moderated by the KI:edu.nrw project the dialog on this is currently being conducted.
An open source prototype of a chatbot integrated into Moodle has also already been developed and put into operation here in the Moodle.nrw project, in cooperation with the KI:edu.nrw project. The basis for this was the LLaMA 2 language model. The infrastructure of the North Rhine-Westphalian High Performance Computing Cluster (Project hpc.nrw) can be used. The aim is to test a highly scaled open-source AI prototype that can be integrated into Moodle, for example, to enable students to interact with course materials via chat. If successful, such a solution could also be implemented for other applications. Ideally, an open source AI that can be adapted for specific purposes can then be made available nationwide.
All questions unanswered?
The issue of providing generative AI or services based on it is an urgent topic for all German universities. The different paths lead to different challenges in terms of costs, data protection and the technical expertise required.
In the short term, it seems unavoidable to use the services of commercial providers. In this context, a close cross-university exchange is recommended so that not every university has to clarify identical questions.
However, if the universities want to have a realistic chance of finding a non-commercial solution, they should start working on it now. This is also not a task for a single university, but requires cooperation across universities – at least at state level, but possibly beyond.
It is likely that the use of AI tools at German universities will result in the coexistence of commercial and open source solutions, from European and non-European providers. Science and politics must now see it as a highly relevant joint task to enable this coexistence and ensure access to language models.
Authors
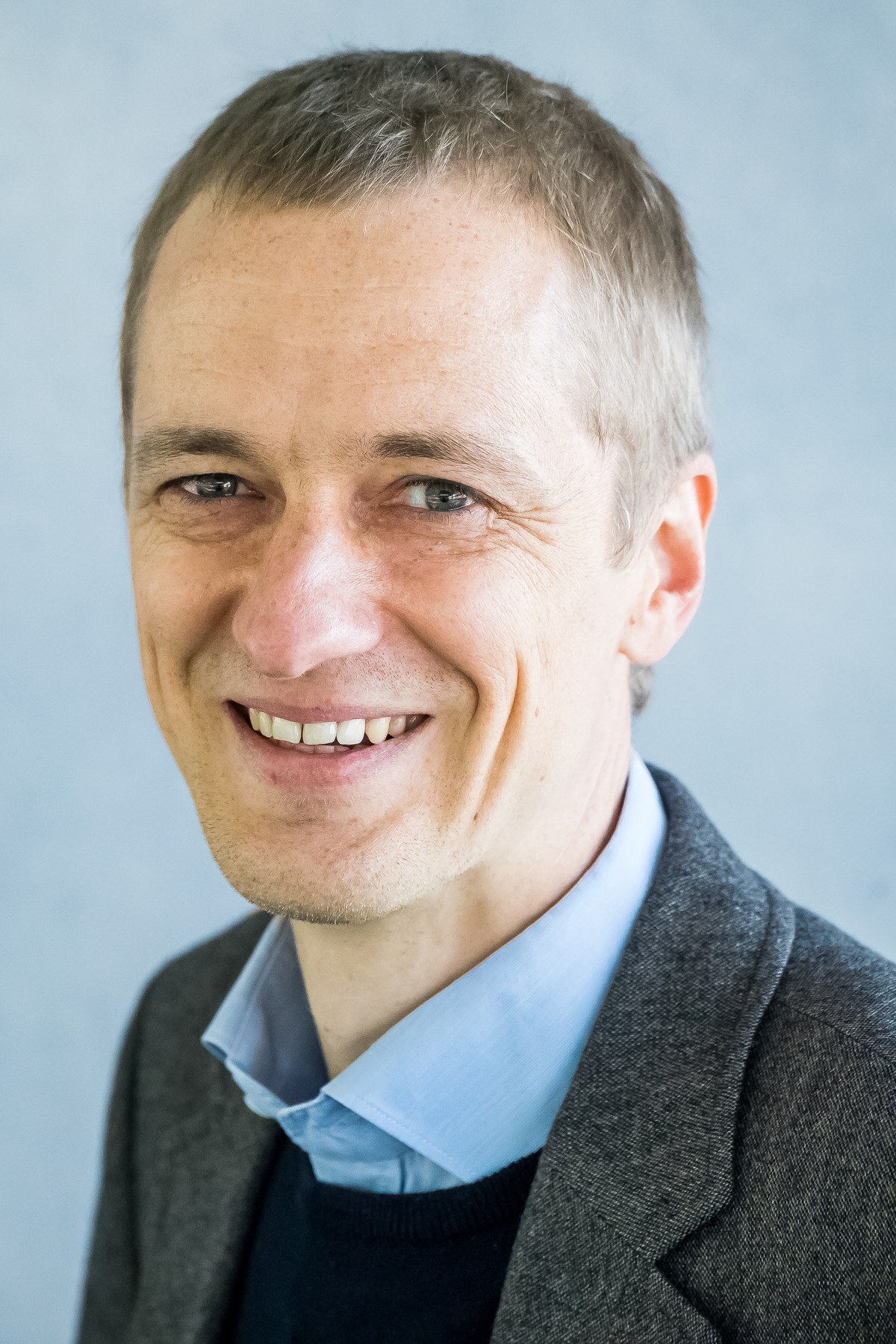
Dr. Peter Salden heads the Center for Science Didactics at Ruhr-Universität Bochum. Within this framework, he is responsible for projects on topics such as artificial intelligence, learning analytics, internationalization, open education and education for sustainable development. Dr. Peter Salden is a member of various advisory boards and committees related to education in the university context.
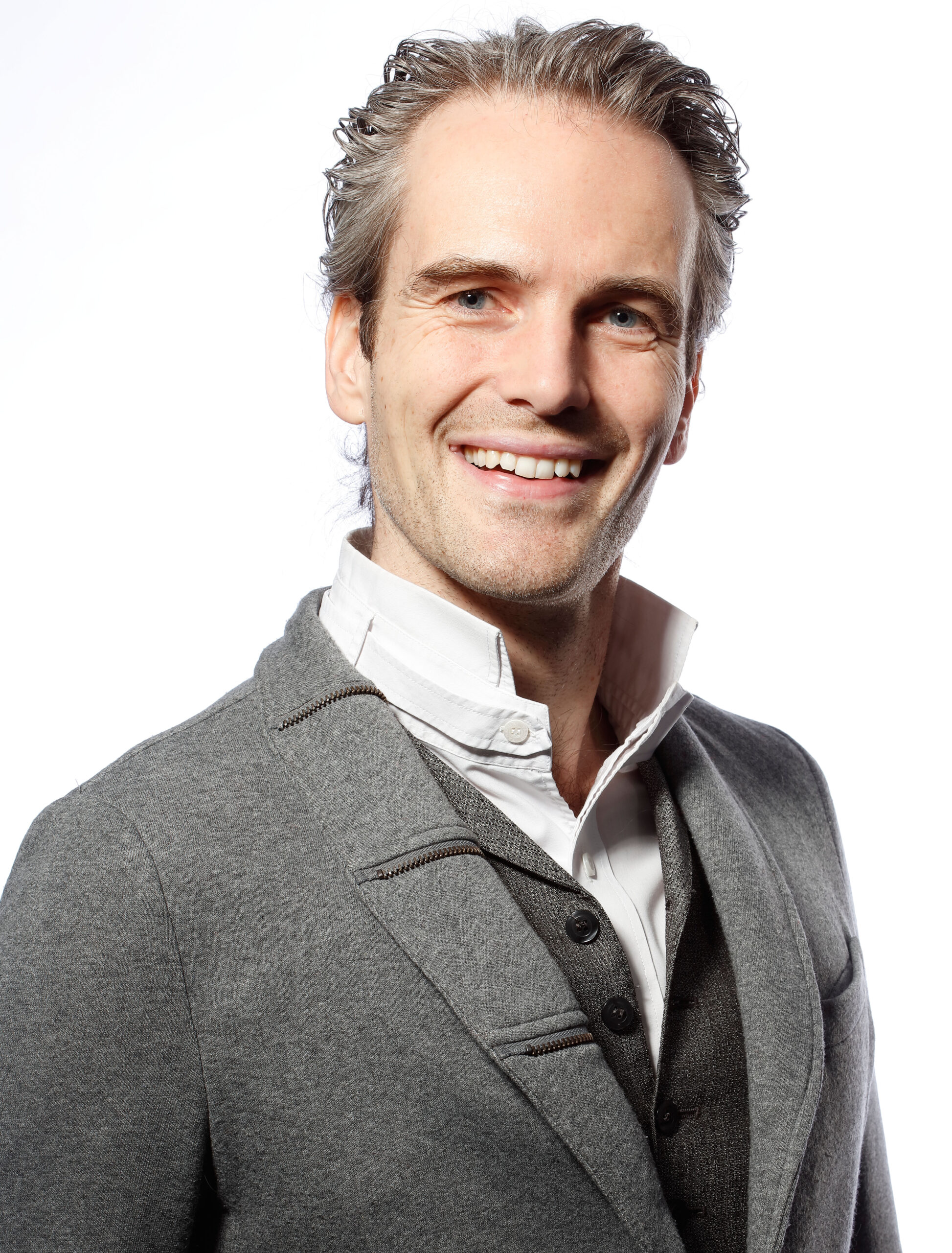
Dr. Malte Persike studied psychology and is currently the scientific director of the Center for Teaching and Learning Services (CLS) at RWTH Aachen University. His research interests include evidence-based impact research in higher education teaching and learning analytics to optimize teaching/learning processes. He is an expert in digital teaching, learning and testing as well as in the data-driven improvement of learning contexts. In 2012, he was awarded the ars legendi prize for outstanding achievements in teaching in the social sciences.
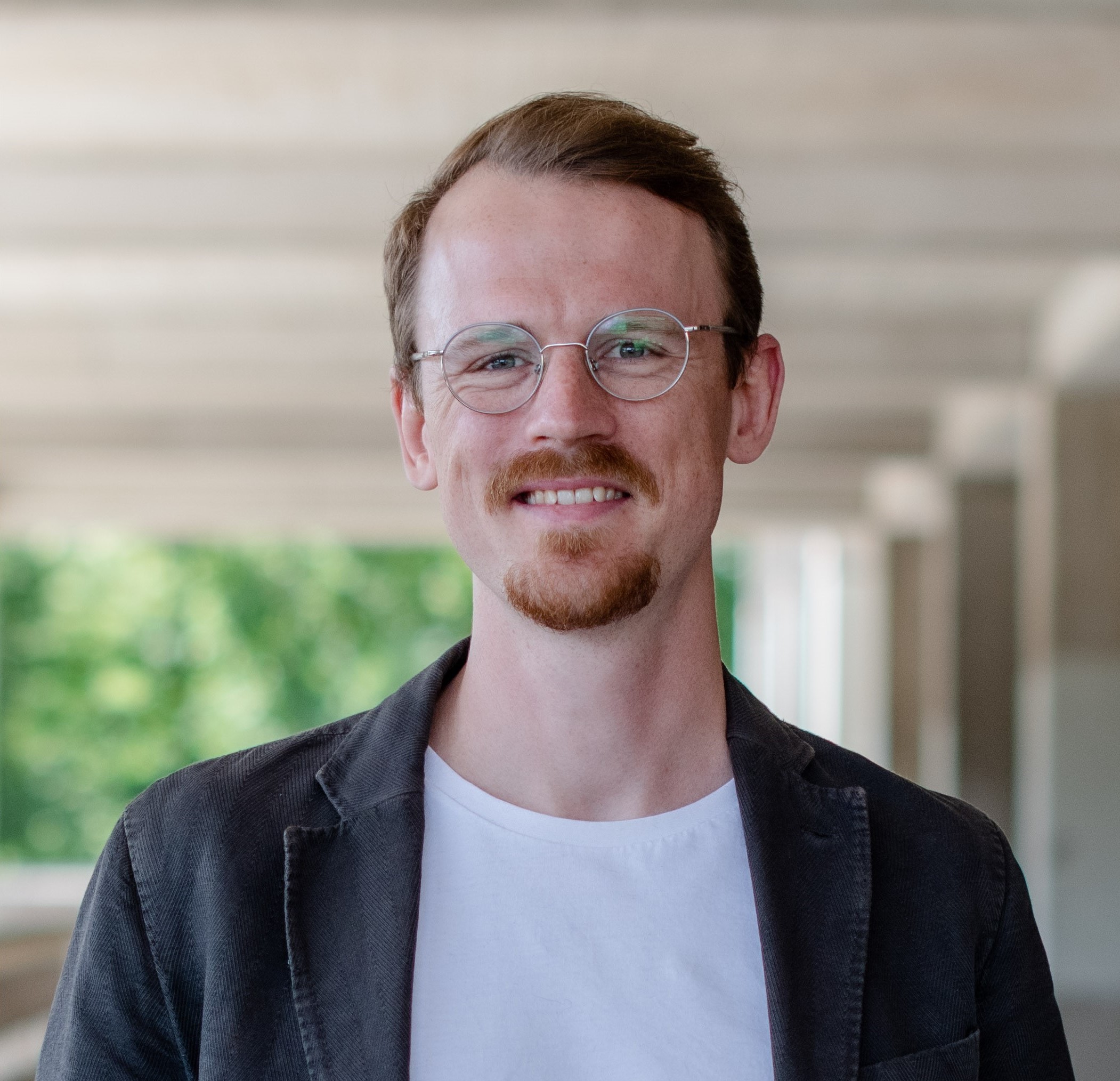
Jonas Leschke is head of the staff unit for strategic teaching projects at the Center for Science Didactics at Ruhr-Universität Bochum.
After completing his teacher training for vocational colleges in the subjects of mechanical engineering and mathematics at the University of Paderborn, he worked in various roles in the field of higher education didactics. The main topics were the professionalization of university lecturers, quality development of university teaching, project-based laboratory internships in teacher training, scholarship of teaching and learning as well as artificial intelligence and learning analytics in university teaching.
How feasible is it for universities in Germany to develop and operate their own AI models for generative AI applications, and what are the potential advantages and disadvantages?Regard Telkom University