Who Is Leading Whom in Science in the Age of Artificial Intelligences?
Who Is Leading Whom in Science in the Age of Artificial Intelligences?
21.10.21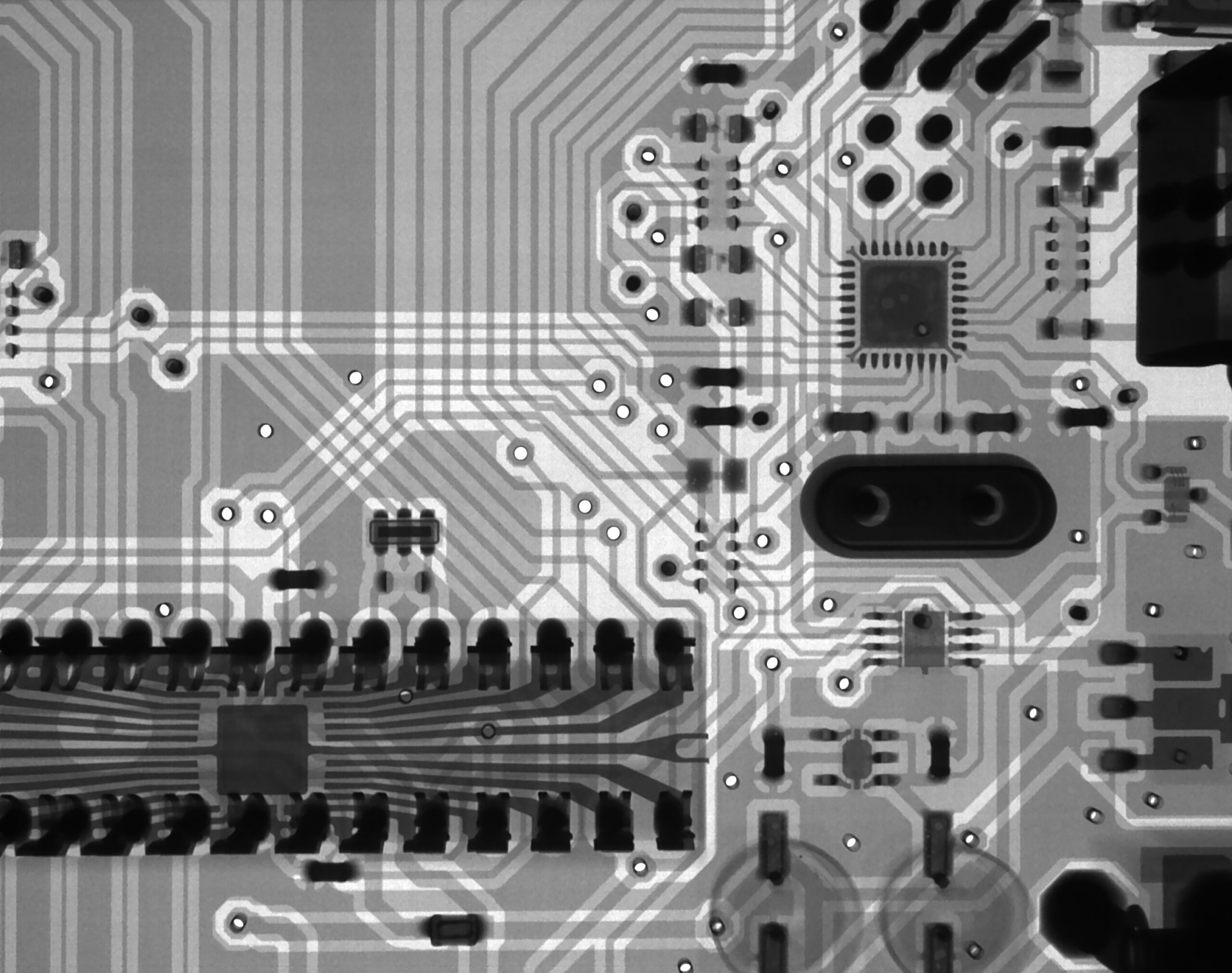
“Leadership is a process by which a person or group influences the behavior of others. In this sense, it is associated with authority. Humans want to be the strongest. While they are currently physically stronger than most other creatures on Earth due to their ability to use tools, this advantage is rapidly diminishing as artificial intelligence advances and robots become more powerful. Humans are also more intelligent than other creatures, but in recent years, artificial intelligence has become smarter and it is likely that artificial intelligence will be much smarter than humans in the future. However, even if they don’t have greater physical or intellectual abilities compared to humans, robots will still be able to take over because humans’ desire for personal power will lead them to make mistakes.”
In order to prevent this cited scenario designed by an artificial intelligence (AI), a normative orientation for the productive cooperation of humans and machines is needed that neither exaggerates the associated dystopian dangers nor naively exaggerates the supposed benefits. So far, however, such an orientation has been lacking, which on the one hand specifies how and for what purpose AIs may be used in the scientific process, and on the other hand defines clear responsibilities. Those values that have guided the scientific enterprise for almost 2500 years are suddenly no longer (easily) adaptable. The traditional values of reliability, honesty, respect, and accountability (ALLEA 2018) are designed for humans as the sole originators of knowledge, using machines at best as a means to an end. Artificial intelligences, however, independently generate knowledge to a degree that exceeds human capability, as DeepMind’s AlphaFold 2.0 artificial intelligence has impressively demonstrated. Or they generate results that are at least indistinguishable from human products, as the first paragraph above shows. Normative categories such as honesty and respect, however, cannot be algorithmized due to their semantic complexity and depth of meaning and thus remain meaningless for artificial intelligences.
Therefore, there is essentially agreement on two things in the discourse: first, that AIs must not assume responsibility for themselves, but that this must always lie with humans (Université de Montréal 2018), and second, that this can no longer be shifted solely to the end user of the AI, who in the rarest cases knows how the AI works and thus cannot provide any accountability for the results generated by means of AI. There are no answers as to how AIs may be used in the scientific process and who is ultimately responsible for what. This is particularly dramatic because this is not a fictitious future scenario, but AIs are already fundamentally integrated in education (Chen et al. 2020) – often enough unnoticed, as in translation or office programs – and there is also a strong political will to push this further (GWK 2020). In this context, the existing lack of orientation with regard to a “correct” use of AI increases the attractiveness for misuse both among students (Weßels 2020) and on the side of researchers (Else & Van Noorden 2021, Lahrtz 2021). So what needs to be done to exploit the as yet incalculable potential of hybrid intelligence (Dellermann et al. 2019), which arises from the interaction between humans and machines, for research as well, but without opening the floodgates to the opportunities for misuse that this opens up at the same time?
In our opinion, two dialogs are urgently needed here – within science and between science and society – if science is to retain its claim to value-based orientation for society. On the one hand, there is the discourse on guidelines and values for the use of AI-based applications. While there are already extensive debates both about integrity in scientific practice (Miller et al., in press) and in the development of artificial intelligences (European Commission 2021; Université de Montréal 2018), the question of the appropriate use of artificial intelligences in scientific practice remains a blind spot, but one that is of incalculable importance, especially for scientific practice. Since traditional values do not seem to apply here, there is certainly some revolutionary potential in this for scientific practices such as the obligation to label one’s own thoughts, not those of others (Wilder et al. 2021).
On the other hand, the discourse must be conducted about who assumes responsibility for what in the complex human-machine interactions. In a first step, we have differentiated between four groups of people:
-
1. The “Creators” develop algorithms for a program, create and manage the reference data corpus, test the software, monitor the system, etc.
2. The “Tool Experts” select suitable AI applications and implement and configure them for their own organization.
3. The classic “Users” can be distinguished between
a. “Producers”, who use the AIs specifically to produce results and
b. “Consumers” who consume, distribute and comment on AI-generated results.
4. The “Affected Persons” are in the widest sense affected by AI-generated content, but without being aware of it.
With this first distinction, we want to open the discourse. The actual work is still to be done, in which, on the one hand, the groups of people must be further differentiated and, on the other hand, it must be negotiated what each group should and can ultimately take responsibility for.In this way, transparency and clarity can be created for each individual who uses AI-based applications for scientific practices. This creates an orientation that puts the potential of hybrid intelligence at the service of the community without the risk of people losing leadership.
The article is loosely based on Wilder et al. 2021. The first paragraph was generated by the app PhilosopherAI and the input “Who leads in the age of AI? The human or the machine?” and “Will there be a trial of strength and a mutual claim of leadership between humans and artificial intelligence in the future?”. The translation was done with DeepL.
Quellen
ALLEA – All European Academies (Hrsg.). (2018). Europäischer Verhaltenskodex für Integrität in der Forschung. http://www.allea.org/wp-content/uploads/2018/06/AL-LEA-European-Code-of-Conduct-for-Research-Integrity-2017-Digital_DE_FINAL.pdf
Chen, X., Xie, H., & Hwang, G.-J. (2020). A multi-perspective study on Artificial Intelligence in Education: Grants, conferences, journals, software tools, institutions, and researchers. Computers and Education: Artificial Intelligence, 1, 100005. https://doi.org/10.1016/j.caeai.2020.100005
Dellermann, D., Ebel, P., Söllner, M., & Leimeister, J. M. (2019). Hybrid Intelligence. Business & Information Systems Engineering, 61(5), 637–643. https://doi.org/10.1007/s12599-019-00595-2
Else, H., & Van Noorden, R. (2021). The fight against fake-paper factories that churn out sham science. Nature, 591(7851), 516–519. https://doi.org/10.1038/d41586-021-00733-5
Europäische Kommission (Hrsg.). (2021). Vorschlag für eine Verordnung des europäischen Parlaments und des Rates zur Festlegung harmonisierter Vorschriften für künstliche Intelligenz (Gesetz über künstliche Intelligenz) und zur Änderung bestimmter Rechtsakte der Union. https://eur-lex.europa.eu/resource.html?uri=cellar:e0649735-a372-11eb-9585-01aa75ed71a1.0019.02/DOC_1&format=PDF
GWK – Gemeinsame Wissenschaftskonferenz (Hrsg.). (2020). Bund-Länder-Vereinbarung gemäß Artikel 91b Absatz 1 des Grundgesetzes über die Förderinitiative „Künstliche Intelligenz in der Hochschulbildung“ vom 10. Dezember 2020. https://www.gwk-bonn.de/fileadmin/Redaktion/Dokumente/Papers/BLV_KI_in_der_Hochschulbildung.pdf
Lahrtz, S. (2021). Forscher decken auf: Hunderte wissenschaftliche Veröffentlichungen wurden durch eine «dumme» künstliche Intelligenz geschrieben. https://www.nzz.ch/wissenschaft/neue-woerter-texte-vermischen-bilder-klauen-ld.1642192?utm_source=pocket-newtab-global-de-DE
Miller, K., Valeva, M., & Prieß-Buchheit, J. (Hrsg.). (in press). Verlässliche Wissenschaft. Bedingungen, Analysen, Reflexionen. wbg.
Université de Montréal (Hrsg.). (2018). Montréal Declaration for a Responsible Development of Artificial Intelligence. https://5dcfa4bd-f73a-4de5-94d8-c010ee777609.file-susr.com/ugd/ebc3a3_506ea08298cd4f8196635545a16b071d.pdf
Weßels, D. (2020). Zwischen Original und Plagiat. https://www.forschung-und-lehre.de/management/zwischen-original-und-plagiat-2754/
Wilder, N., Weßels, D., Gröpler, J., Klein, A., & Mundorf, M. (2021). Forschungsintegrität und Künstliche Intelligenz mit Fokus auf den wissenschaftlichen Schreibprozess. Traditionelle Werte auf dem Prüfstand für eine neue Ära. In K. Miller, M. Valeva & J. Prieß-Buchheit (Hrsg.), Verlässliche Wissenschaft. Bedingungen, Analysen, Reflexionen (S. 523). wbg. https://files.wbg-wissenverbindet.de/Files/Article/ARTK_ZOA_1025976_0001.pdf