Digital and intellectual sovereignty in the age of AI: An assessment for the higher education sector
Digital and intellectual sovereignty in the age of AI: An assessment for the higher education sector
06.02.25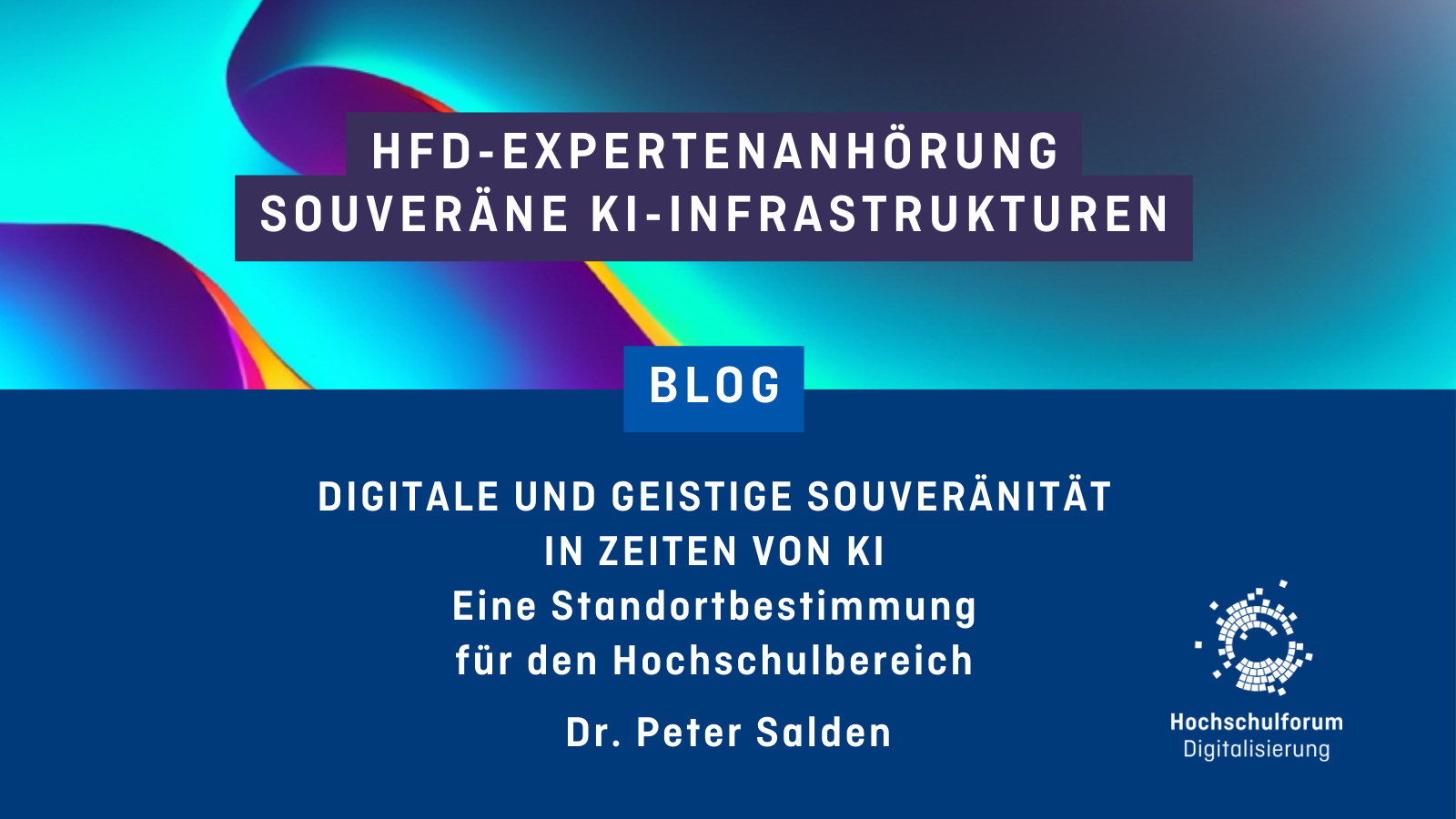
To what extent can universities still be considered digitally sovereign in the age of AI? How dependent are they on commercial AI providers, and what can they shape themselves? And: do universities also need to take language models into their own hands to ensure independent scientific thinking? Dr. Peter Salden from the Centre for Science Didactics at Ruhr University Bochum provides an overview and assesses the current situation. The text was written on the basis of his contribution to the expert hearing Digital Sovereignty, which was Hochschulforum Digitalisierung organized in Cologne in December 2024.
Digital sovereignty in the age of AI
The topic of digital sovereignty has occupied universities for much longer than the topic of artificial intelligence (AI). To what extent can universities become independent of commercial providers? Can they program software themselves, maintain it themselves and store data on their own servers? When it comes to learning and campus management, they have shown that this is certainly possible. However, other examples, such as the use of Office products, also showed limitations. And then came AI.
A leap back to the year 2023: Members of German universities get to know the world of generative AI – usually via ChatGPT. They register on the OpenAI website with their personal data and use the chatbot offered by the company. The resulting data is processed on the company’s servers. Experiments are not always meaningful, and users and institutions sometimes lack an understanding of the technology. It’s the Wild West of the AI age in universities.
What would digital sovereignty mean in comparison? For individual users, it would mean using AI in a way that is aware of how it works, the opportunities and limitations of this technology; in other words, using it on the basis of so-called “AI literacy”. From the perspective of an institution, digital sovereignty would also mean not being dependent on commercial providers for an AI service and instead holding technical elements in their own hands. In addition, digital sovereignty would also mean having defined institutional framework conditions for use – legal rules, but also strategies for didactic use and staff training, for example.
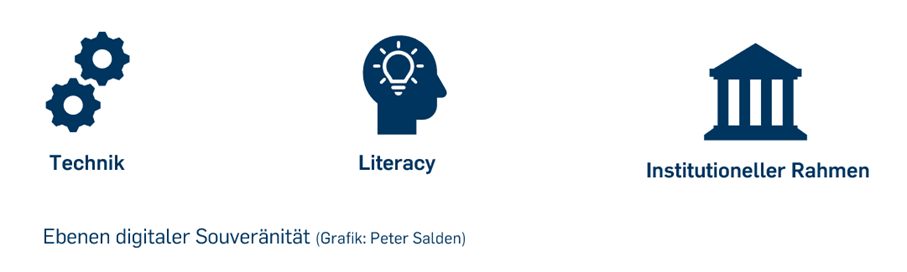
In 2024, universities have made great strides towards AI sovereignty. But there are challenges – especially in the technical field.
Technical components
From a technical perspective, AI provision involves different components that universities can either obtain from commercial providers or develop and operate themselves. The closest thing to the user is a webinterface that offers a specific service. For example, this could be a homepage that makes a general chatbot accessible. However, other services are also conceivable, such as tools for providing feedback on written work. In the educational context, it is very important that the service – the chatbot, for example – responds as accurately as possible. Many universities are therefore working on solutions for so-called “Retrieval Augmented Generation”(RAG) systems. This refers to a technical process in which answers are based on predefined facts. Whether with or without RAG, the core of the system is then the language model that processes a query. In the end, all data must be stored on servers(hosting). The interfaces between the components are also important in the interaction.
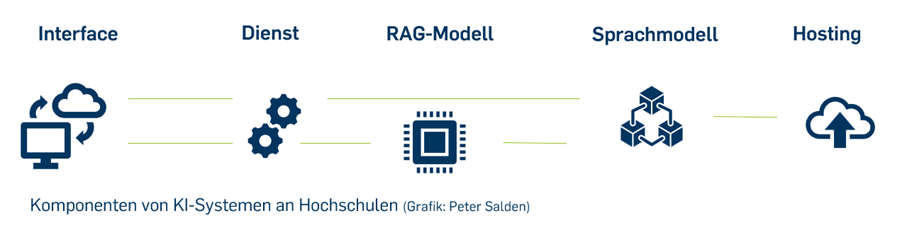
Universities can theoretically take each of these components into their own hands – and in some cases are already doing so. The following can be said with regard to the individual components:
The method of making language models from OpenAI (and other providers) accessible via a dedicated web interface using an application programming interface (API) is currently particularly widespread at German universities – usually for a general chat service. In contrast to using the models directly via the commercial websites, the personal login data remains at the university in this procedure. When chatting, only the chat requests (prompts) and general institutional data are passed on to the external service providers – a step forward for data protection.
The challenge is that indirect access does not automatically offer all the functions that users are familiar with from ChatGPT, for example. This is because all these functions have to be “recreated” for the user’s own interface, which means considerable effort and a constant need for further development.
Special services (such as feedback tools for texts) are now also being developed at universities on the basis of available models. Technically, this can often be achieved with reasonable effort. However, questions of integration into the existing system landscape must also be considered, for example connecting the service to the university’s identity management system or integrating it as a plugin into the learning management system. The result can be a small “AI ecosystem” of different services that are available to teachers and students at a university.
The topic of RAG is also currently occupying many universities. The focus is on the basic scenario of linking chatbots to a clearly defined pool of information for their answers (e.g. a lecture script in pdf form). Students should then receive answers based on precisely these verified facts when interacting with the bot. Here, too, it is becoming apparent that universities can certainly provide appropriate solutions. However, it is also clear that there is still a need for research in this area (for example, so-called “chunking”) to ensure that the systems function as reliably as possible – and that despite the possibility of increasing fact accuracy, there will not be a complete guarantee for correct output of language models.
Training your own large language model (LLM) is a task that cannot be accomplished by a single university due to the resources required (expertise, training data, computing power, etc.). However, it is possible for universities to use open source models, which can be stored on their own servers and whose functionality can be influenced (fine-tuning). Concrete services based on open source models (especially those that have been adapted in-house) are still rare. However, it is noticeable that interest in this direction is growing and that universities have the expertise to do this.
If universities are interested in using open source models for permanently accessible services (such as a chatbot in the student advisory service), the question arises as to where the service and model can be hosted. This is a particular problem when many thousands of people at a large university need to be able to use a service around the clock. The computing power required for this is expensive and should be utilized as well as possible, which speaks against local hosting.
To solve this problem, many universities are now using the option of obtaining open source models via external hosting from commercial service providers (e.g. Microsoft Azure Cloud) or from public institutions (e.g. GWDG in Göttingen). The use of cross-university High Performance Computing (HPC) clusters is also coming into focus. However, this infrastructure is often neither designed for “round-the-clock” operation nor for a high number of small-scale user requests from the universities, so there are also hurdles to overcome with regard to HPC use.
Cooperation
In theory, universities certainly have the know-how and resources to increase their level of digital sovereignty in the age of AI. However, it is also clear that achieving this for all individual components of an AI system is no small feat and can hardly be accomplished by individual universities – especially smaller ones. It doesn’t make sense to go it alone anyway, because while many people work on one problem in large tech companies, at many German universities individual people are still too often working on the same problem in isolation. Universities are not necessarily weak – but digital sovereignty in the age of AI can only be achieved by joining forces.
In fact, collaborations are already emerging at all points in the component chain. Take interfaces for chatbots, for example: cooperation projects such as the state project KI:Connect.nrw have been formed here, through which a user interface for a chatbot is made available open source for installation (or state-centric use) to all interested universities. It is to be expected that a limited number of such jointly developed interfaces will be in operation at German universities in the future.
The same could happen with other services that are of interest to many universities. New communities are not always necessary for this: Important anchor points for collaboration on AI provision are also existing communities in the open source sector, such as the communities of learning management systems. For example, an intensive exchange is already taking place here for the integration of RAG-based chatbots in Moodle.
Another example of cooperation is hosting: as already mentioned, solutions are already being designed and implemented across universities. Universities from all over Germany can access open source models that are operated in the federally funded AI service center KISSKI, which is also run by the publicly funded GWDG. The Open Source-KI.nrw project in North Rhine-Westphalia is also an example of efforts to open up the state’s central HPC infrastructure to all universities in a federal state. It is to be expected that this trend will continue, i.e. that universities in large federal states will jointly access state-owned computing capacities and that small federal states in particular will use infrastructure from other states for hosting.
The breakdown of the AI provision chain into components outlined above makes it clear that collaboration is not always and everywhere necessary – as specific services, for example, can also be developed at a single university. Nevertheless, a partially coordinated approach to software development and hardware use is desirable so that universities can use “AI-sovereign” solutions flexibly.
An underestimated problem: mental sovereignty
Let’s take a look at the overall picture after determining the current situation: assuming smart cooperation, universities could provide interfaces, services and RAG models themselves in the future and also solve the hosting problem. They could integrate commercial (proprietary) or open source language models into their infrastructure. However, in order to be able to offer a fully “green”, i.e. digitally autonomous AI infrastructure, there is a gap in the chain: the lack of proprietary language models.
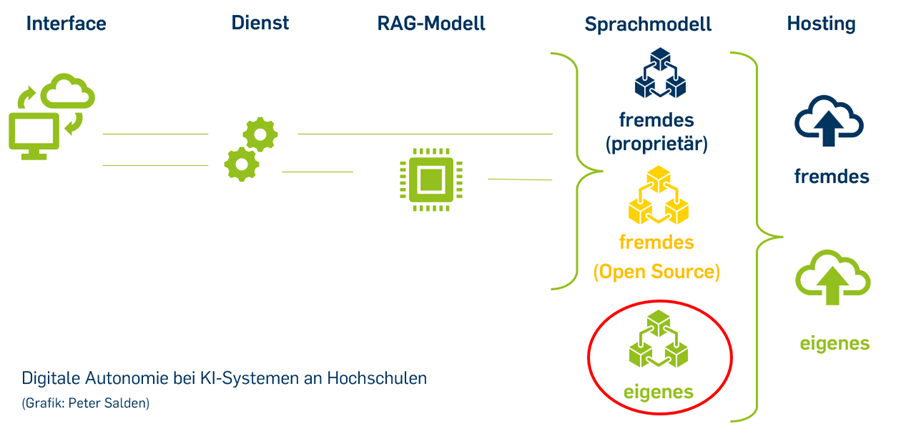
Developing your own language models (especially LLM) remains a challenge that seems almost insurmountable when you consider the high investment costs of commercial models. However, the core of university AI infrastructure always comes from outside as long as there are no in-house developments. Ultimately, this also applies to open source models if they have been trained by a large tech company (such as Llama) and this process is not transparent in every detail.
Why is that a problem? Let’s take another look at everyday life at universities: Students (and academics) are increasingly entering tasks into a chatbot to see how far they can get in solving their problem. The result is then reworked if necessary. This applies not only to the presentation of facts, but also to argumentative tasks. Thinking thus increasingly takes its starting point in the model.
However, the language models on which chatbots and other services are based not only have a problem with the accuracy of their responses. Much less noticed is the fact that they are not neutral in terms of ideology either. However, a Chinese language model is just as fine-tuned in this respect as an American model. Until now, this has hardly been an issue in Germany, as the Western models generally reflected our view of the world well and did not, for example, spout any neo-Nazi slogans. But will it stay that way?
The elections in the US have shown how strongly social media can influence thinking and that the owners of social media channels are willing to use their power over tools and algorithms. So what happens when language models become more and more part of our everyday lives and students and academics alike base their arguments on answers from language models? For example, what will academic texts on gender research look like if Elon Musk’s chatbot “Grok”, advertised as “anti-woke”, is used to write them? What will works on Chinese history look like if they are generated by a Chinese chatbot?
These problems show that digital sovereignty is not just a question of data protection and independence from commercial cost models, but that the core issue of digital sovereignty in the age of AI is intellectual sovereignty. Language models entail a considerable risk of subliminal manipulation. They affect the freedom of thought, and therefore the freedom of science. The question of language models is therefore the key question of digital sovereignty. And this is precisely the weak point (not only) of universities in their efforts to establish this sovereignty on their own.
In search of solutions
All in all, German universities have already made good progress on their way to building sovereign AI infrastructures. They have shown that a lot is possible – and that is cause for optimism. The cooperation that is emerging in many areas is also positive. However, the important question of the extent to which the language models themselves can be controlled by universities remains unanswered.
The situation does not have to be hopeless with regard to the latter point either. No, universities will not be able to train and develop their own language models with resources comparable to those of the big tech companies. However, they can focus even more strongly on the further development of open source models, on the adoption of models developed in Europe, on cooperation with commercial European providers or on the development of small language models. Research, development and funding policy should focus more on this. It can be helpful to consider that the wide range of functions of commercial large language models is not always necessary for university applications in teaching and learning, research and administration, but that specialized applications can also perform very well for their purpose with fewer resources. The example of the Chinese DeepSeek model is also encouraging in that it appears to have succeeded in training a powerful LLM with comparatively little effort.
Ultimately, however, the problem of models shows that digital sovereignty should not be thought of solely on a technical level. Users of AI applications at universities must understand the functional principles and risks of the systems, and universities must set a clear framework. Technology, literacy and institutional framework conditions – these are the three fields of action for digital sovereignty in times of AI that need to be worked on now, often across universities.